In 2024, AI is no longer a luxury, it’s a necessity. Companies that effectively implement AI solutions are seeing significant gains, with McKinsey reporting that AI adoption has more than doubled in the last five years.
Furthermore, businesses integrating AI at scale are 25% more likely to outperform their competitors in key financial metrics, such as profit margins and productivity. These statistics highlight AI’s potential to transform industries, but as more companies rush to adopt AI, understanding AI development costs have never been more important
The question isn’t whether to adopt AI, but how much it will cost and whether the investment will generate tangible returns. Misjudging these costs can lead to wasted resources and missed opportunities.
For executives, staying on top of these pricing trends is crucial for making strategic, data-driven decisions in the fast-evolving AI landscape.
The True Cost of Implementing AI & Data Solutions in 2025 - A Comprehensive Guide For Business Leaders
Types of AI Implementations
AI comes in different forms, each offering distinct capabilities and corresponding pricing structures.
To fully understand the cost of AI development, it’s essential to differentiate between the types of AI that businesses can implement, as the price can vary significantly depending on the complexity and scope of the solution.
Let’s dive into the main categories:
Narrow AI (Weak AI)
Narrow AI, also known as Weak AI, is the most commonly used form of artificial intelligence today. It is designed to perform specific, limited tasks, making it highly practical for businesses looking to solve particular problems without extensive investment.
Examples of Narrow AI include chatbots, personalized recommendation systems, and predictive analytics tools. These applications are widely adopted in customer service, marketing, and operations due to their targeted approach.
Narrow AI is typically more cost-effective than more advanced AI systems because it requires less computational power and narrower data sets. Businesses can implement Narrow AI with lower initial investment, especially if they use pre-built models or cloud-based solutions.
According to Netflix, its recommendation engine drives over 80% of the content users watch, significantly improving engagement while reducing churn rates.
General AI (Strong AI)
Unlike Narrow AI, General AI (also referred to as Strong AI) aims to simulate human intelligence across a wide range of tasks, not just specific applications.
While this level of AI development remains largely theoretical today, the potential of General AI has captured the attention of industry leaders and researchers. The concept behind General AI is to create a machine that can understand, learn, and apply knowledge in various domains, much like a human.
The development of General AI requires significant research and investment, often amounting to millions of dollars in R&D. The complexity of General AI systems means they are far from being commercially viable for most businesses today, and the costs are prohibitive for all but the most advanced research labs.
Machine Learning vs. Deep Learning
Machine Learning (ML)
Machine Learning is a branch of AI development that allows systems to learn from data and improve over time without being explicitly programmed. It is used in various business applications, such as customer segmentation, fraud detection, and process automation.
Machine Learning models rely heavily on data and algorithms to identify patterns, make predictions, and optimize operations.
The cost of implementing ML largely depends on the quality and volume of data, as well as the infrastructure required to process it. While Machine Learning can be implemented at a relatively moderate cost, businesses must invest in data cleaning and model training to ensure accurate results.
According to a Gartner report, ML is one of the fastest-growing AI segments, with 45% of companies expected to implement it by 2025.
Deep Learning (DL)
Deep Learning, a more advanced subset of Machine Learning, uses neural networks with multiple layers to analyze large datasets and handle more complex tasks like image recognition, speech processing, and autonomous decision-making.
Deep Learning excels in areas requiring vast computational power and large amounts of labeled data.
The costs of Deep Learning are higher due to its reliance on advanced hardware, such as GPUs (Graphics Processing Units), and the need for significant computational power.
Moreover, the data requirements for Deep Learning models are immense, driving up storage and data preparation costs. The payoff, however, is that Deep Learning can unlock advanced capabilities that simpler models cannot achieve.
Factors Influencing AI Development Costs
The costs of implementing AI vary greatly depending on several key factors. For businesses understanding these factors is essential to making strategic decisions about AI investments.
Below are some of the most significant factors influencing the price of AI developmen
Data Requirements
Data is the fuel for any AI development system, and the quantity, quality, and type of data required will significantly impact pricing. AI models rely on vast amounts of high-quality data to perform effectively.
Data Acquisition
Acquiring the right data can be expensive, especially if you need to purchase proprietary datasets or invest in data-gathering systems. Companies operating in data-sensitive industries like healthcare or finance may also face additional costs for ensuring data security and compliance.
Data Preparation
Raw data is rarely ready for use in AI models. It needs to be cleaned, labeled, and processed. This can be a time-consuming and costly task, especially if the data needs to be more structured and complete. Data scientists and engineers are typically required to handle these tasks, and their expertise comes at a premium.
Storage and Management
AI development models often require significant storage space for both the data they process and the models themselves. Cloud-based solutions offer flexible pricing but can become expensive over time as data volumes increase. On-premise data storage might have higher upfront costs but can provide more control over long-term expenses.
Customization Level
The extent to which an AI solution is tailored to specific business needs can also greatly influence the overall pricing.
There are generally two approaches to AI implementation: off-the-shelf solutions and fully customized systems.
Off-the-Shelf AI
Pre-built AI models, available through platforms like AWS, Google Cloud, or Microsoft Azure, provide a more affordable entry point. These solutions are scalable, easy to deploy, and require little customization.
However, they may not be perfectly suited to a business’s unique needs, which could limit their effectiveness.
Custom AI Solutions
Businesses seeking to solve more complex problems or gain a competitive edge often invest in fully customized AI systems. Customization requires a greater investment, including hiring specialized AI developers and data scientists, and can significantly increase the cost of development.
However, the benefits of tailored AI can outweigh the upfront expenses by providing highly optimized and scalable solutions.
Talent Acquisition and Expertise
One of the most significant drivers of AI pricing is the cost of acquiring talent. The demand for AI specialists like data scientists, machine learning engineers, and AI developers continues to surge, driving up salaries and making talent acquisition one of the largest expenses for companies looking to implement AI.
Hiring AI Talent
AI expertise is highly specialized, and recruiting top-tier talent comes at a premium. According to PwC, the global shortage of AI professionals has led to rising salaries and competitive hiring markets, particularly for roles in machine learning and data science.
Companies must factor in not only the cost of hiring but also training and retaining AI specialists.
Outsourcing AI Development
Some businesses choose to outsource AI development to external vendors or AI service providers to reduce the costs associated with in-house talent. While outsourcing can provide a cost-effective solution, it may also come with risks related to control, data privacy, and long-term scalability.
Companies need to carefully weigh the benefits and drawbacks of outsourcing against building internal AI capabilities.
Breaking Down AI Development Costs
When it comes to AI pricing, the real challenge is understanding how costs are distributed across various stages of implementation. These costs are not one-time investments; they stretch across development, deployment, and long-term maintenance.
Below is a detailed breakdown of the core expenses involved in AI implementation:
Initial Setup Costs
1. Software and Platform Selection
One of the first decisions businesses must make is which platform or software to use. Cloud providers like AWS, Google Cloud, and Microsoft Azure offer AI development services on a pay-as-you-go basis.
The cost structure typically includes charges for computational power (CPU/GPU), storage, and data processing.
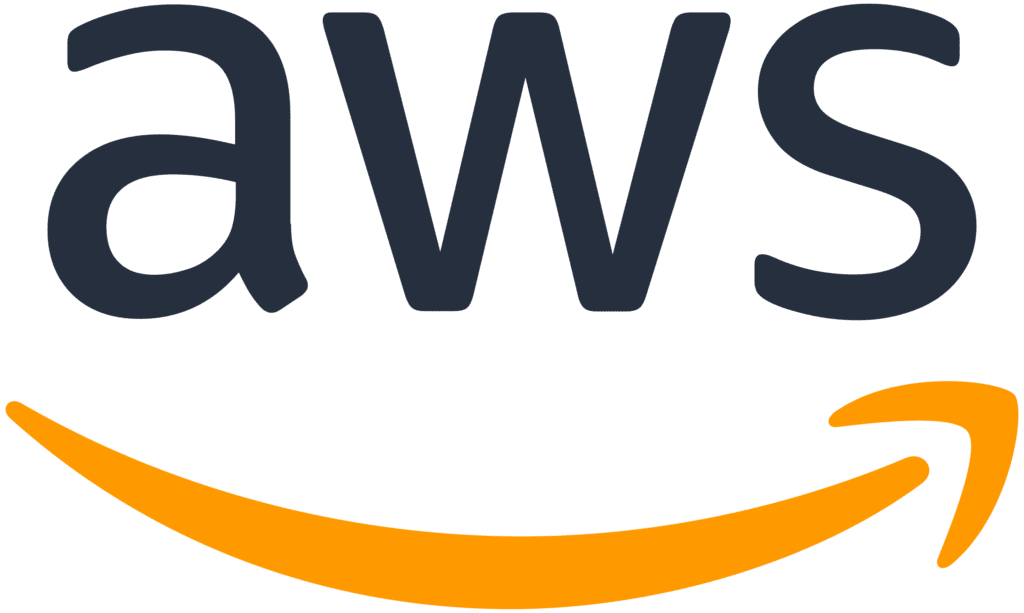
AWS
AWS charges for SageMaker, its machine learning service, starting at $0.065 per hour for basic instances. For high-performance, GPU-based computing, the cost can jump to $3–$4 per hour depending on the instance type and region.
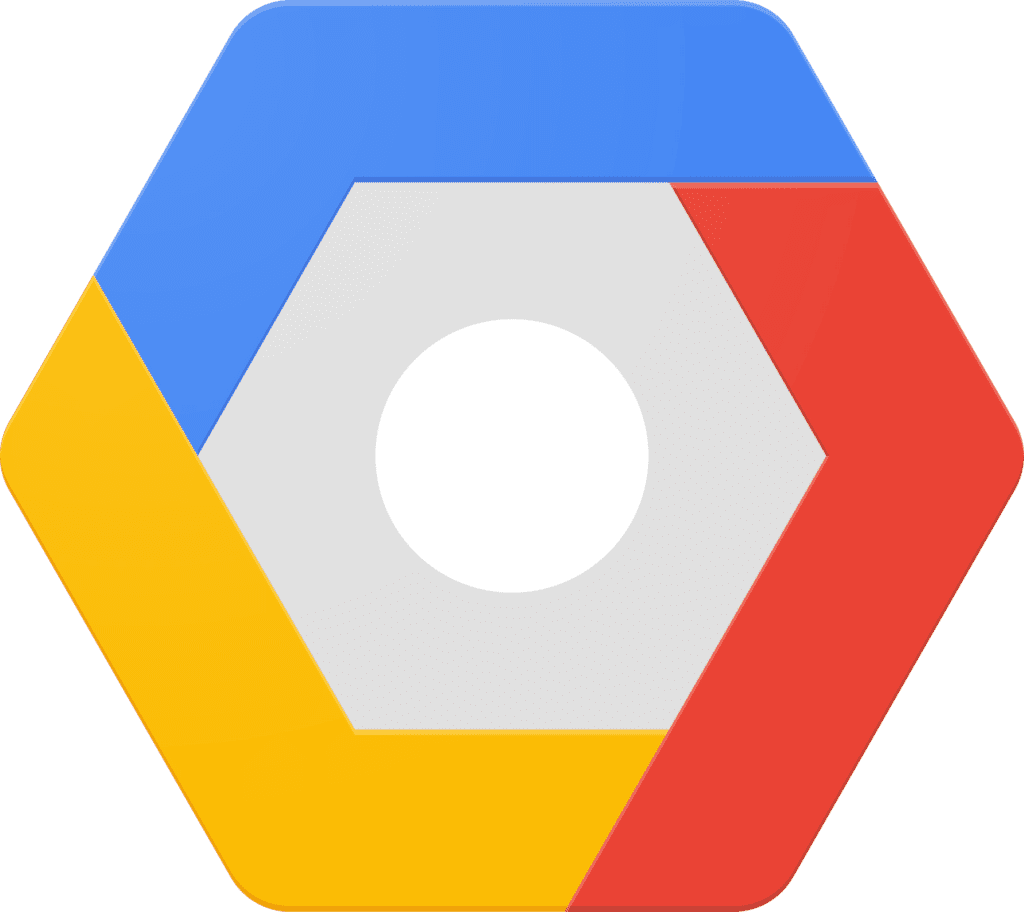
Google Cloud
For Google’s Vertex AI, training models on CPUs starts at $0.058 per node hour. For advanced GPUs, costs rise to $2.76 per node hour for A100 GPUs, commonly used in complex AI projects.
2. Data Acquisition and Preparation
Data is the cornerstone of any AI implementation, but preparing it for use comes with significant costs. The expense of data acquisition, cleaning, and labeling can account for up to 80% of the total project budget.
Here’s a look at the specific costs involved:
Proprietary Data Acquisition
Purchasing external datasets can cost between $1,000 to $100,000+ per dataset depending on the industry.
Data Labeling
Companies like Scale AI or Labelbox provide human-annotated data at prices ranging from $0.05 to $0.50 per label, with costs scaling significantly based on the complexity of the dataset.
Data Cleaning
Hiring data engineers to clean and preprocess the data can cost companies around $80,000 to $120,000 annually per employee.
Ongoing Operational Costs
1. AI Maintenance and Model Updates
AI development requires continuous updates and improvements, especially as new data becomes available. Maintenance costs include monitoring the model for accuracy, retraining, and ensuring that the system is operating efficiently.
Model Retraining
The cost to retrain a machine learning model can range from $10,000 to $50,000 per iteration, depending on the model’s complexity and the data involved.
Model Monitoring
AI systems need to be actively monitored for accuracy and performance. Hiring dedicated AI specialists or engineers for this task typically costs around $120,000 to $150,000 annually per employee.
2. Cloud and Infrastructure Costs
For AI models hosted on the cloud, ongoing operational costs include cloud storage, compute resources, and network bandwidth. The scalability of these costs depends on the size of the model and the volume of data processed.
Storage Costs
Storing vast datasets and AI models can cost businesses between $0.023 and $0.25 per GB per month on platforms like AWS or Google Cloud.
Compute Costs
Running AI models, especially those using GPU-heavy computations like deep learning, can be expensive. Cloud providers typically charge $1 to $5 per hour for compute time, depending on the resources required. For large models running continuously, this can result in monthly bills upwards of $20,000 to $100,000.
* The prices are subject to change depending on market rates or change in company policies.
Tips for Maximizing Your AI Development Budget
Implementing AI can be a significant investment, but with a strategic approach, you can optimize your budget and maximize the return on investment.
Here are some tips to help you get the most out of your AI budget:
1. Start Small and Scale Gradually
One of the best ways to manage your AI development budget is to start with smaller, targeted projects before scaling up. Rather than jumping into large, complex AI solutions, focus on one or two specific use cases where AI can provide immediate value such as automating customer service or optimizing marketing campaigns.
Launch a small-scale pilot to test the effectiveness of AI in one area of your business. This allows you to evaluate its performance and refine your strategy without significant financial commitment.
By starting with Narrow AI applications, such as chatbots or recommendation engines, you can quickly deploy cost-effective solutions that deliver results and build the case for larger investments later.
2. Leverage Pre-Built AI Solutions
Instead of investing in fully customized AI models, consider using off-the-shelf AI solutions or platforms. Pre-built solutions from providers like AWS, Google Cloud, or Microsoft Azure can help reduce development costs while still delivering powerful capabilities.
AIaaS platforms allow businesses to pay only for the services they need, reducing upfront costs and offering flexible scaling options. These platforms typically offer tiered pricing so that you can align your spend with your current needs.
3. Outsource When Needed
Hiring and retaining a team of AI development specialists can be expensive, particularly in today’s competitive talent market. Outsourcing certain aspects of your AI projects to specialized vendors or consultants can help reduce labor costs while providing access to expertise.
Focus your in-house team on core AI strategies that align with your business goals, while outsourcing non-core tasks like data labeling or model monitoring to external providers.
4. Consider Long-Term ROI
Finally, always assess the long-term return on investment (ROI) when planning your AI development budget. While AI projects may have high upfront costs, they can deliver significant value over time by increasing efficiency, improving decision-making, and creating new revenue streams.
Focus on AI projects that provide clear, measurable outcomes such as cost savings, revenue growth, or enhanced customer satisfaction. This ensures that your AI investment continues to drive value over time.
Key Takeaways to Build a Data Strategy That Works
The journey to implementing AI is a significant but rewarding one. As we’ve explored, AI development costs can vary widely and are influenced by factors like data acquisition, platform choices, and talent requirements.
However, with careful planning and strategic investment, businesses can not only manage these costs but also maximize the returns from their AI initiatives.
Those who invest wisely will not only see significant returns but will also position themselves as leaders in their industries. The key is to balance ambition with pragmatism, ensuring that every dollar spent on AI development is an investment in the future.
By following the tips outlined in this guide, your business can stay competitive, drive meaningful innovation, and harness the full potential of AI, without breaking the bank.
Frequently Asked Questions
What are the main factors influencing the cost of AI development?
AI pricing is influenced by several key factors, including the complexity of the AI solution, data acquisition and preparation costs, platform and software selection, infrastructure needs (cloud or on-premise), and the cost of talent for AI development and maintenance. Custom AI solutions tend to be more expensive than pre-built ones, and ongoing operational costs such as model updates and monitoring must also be considered.
How much does it cost to implement AI for a business?
The cost of implementing AI varies depending on the scope and scale of the project. Small-scale AI projects using off-the-shelf models can start at around $50,000, while more complex, custom-built AI systems can cost upwards of $300,000 or more. Deep learning projects and large-scale AI initiatives may exceed $1 million, especially when factoring in data acquisition and infrastructure costs.
How can businesses reduce AI development costs?
Businesses can reduce AI costs by starting with smaller pilot projects, using pre-built AI solutions like those offered by Google Cloud or AWS, and outsourcing non-core tasks such as data labeling or model monitoring. Focusing on high-quality data over large quantities and utilizing hybrid cloud infrastructure can also help manage long-term operational costs.
Is it better to build a custom AI model or use a pre-built solution?
Choosing between a custom AI model and a pre-built solution depends on the business’s specific needs. Custom models offer more flexibility and can be tailored to meet unique requirements, but they come with higher costs. Pre-built AI solutions are more affordable and faster to implement, making them a great choice for businesses looking to deploy AI quickly and at a lower cost.
What is the ROI on implementing AI development?
The return on investment (ROI) for AI implementation depends on the type of AI solution and how effectively it is integrated into business operations. While AI development can have high upfront costs, businesses can see significant long-term returns through increased efficiency, cost savings, and new revenue opportunities. Measuring the impact of AI on key business metrics is essential for evaluating ROI.