As businesses race to stay competitive in a data-driven world, supply chains face a major challenge: turning vast amounts of scattered data into actionable insights.
The real game-changer? Data integration.
Big data has become the keystone of modern supply chains. The vast streams of information generated by IoT devices, ERP systems, CRM platforms, and external market sources are reshaping how businesses forecast demand, manage inventory, and navigate logistics.
Yet, while the power of big data is undeniable, its true value lies in how effectively it’s integrated across the supply chain ecosystem.
By unifying diverse data sets, companies can leverage AI-driven analytics to transform raw information into predictive insights and actionable strategies. This shift from siloed data to interconnected intelligence enables organizations to anticipate disruptions, optimize delivery routes, and streamline operations with unprecedented precision.
In this world abuzz with data, and AI chasing it on its heels, let us try to explore how big data integration is revolutionizing supply chain performance. We’ll attempt to examine how industry leaders are turning these integrated data flows into competitive advantages, driving efficiency gains, cost savings, and greater agility in their operations.
The True Cost of Implementing AI & Data Solutions in 2025 - A Comprehensive Guide For Business Leaders
The Role of Data Integration in Modern Supply Chains
Data integration in supply chains refers to systematically merging data from various sources into a unified framework that enables comprehensive analysis and decision-making.
In a time dominated by big data, the seamless integration of information across platforms like Enterprise Resource Planning (ERP) systems, Customer Relationship Management (CRM) software, Internet of Things (IoT) sensors, and logistics databases is critical for maintaining operational coherence.
Integrating data is not just combining information, it’s creating a synchronized data ecosystem where insights flow freely across all levels of the supply chain. This interconnectedness allows supply chain leaders to make data-driven decisions with greater accuracy, speed, and foresight.
The Strategic Importance of the Supply Chain
A well-integrated supply chain enables companies to anticipate market trends, reduce operational costs, and respond swiftly to disruptions. As the pace of global commerce accelerates, the need for agile, data-driven supply chains has never been more critical.
Supply chain performance is a key differentiator for industry leaders.
According to McKinsey, companies that leverage integrated data analytics in their supply chain operations achieve a 15-20% increase in inventory accuracy and a 5-10% reduction in logistics costs. These figures highlight the transformative impact of data integration on supply chain efficiency, agility, and resilience.
How Data Integration Enhances Supply Chain Performance
The role of data integration extends beyond data consolidation. It is pivotal to the execution of AI-enhanced analytics that modern supply chains rely on.
When data from every node in the supply chain is integrated, it allows for:
-
Enhanced Demand Forecasting:
Leveraging big data from sales trends, market conditions, and customer behavior to predict demand shifts more accurately and reduce stockouts or overstock situations. -
Real-Time Inventory Management:
Creating a consolidated view of inventory levels across all warehouses, leading to dynamic stock adjustments and automated replenishment systems that minimize holding costs. -
Optimized Procurement Processes:
Using data from suppliers, market rates, and production schedules to make just-in-time procurement decisions, which reduces lead times and enhances supplier relationship management.
By synthesizing data from disparate sources into a cohesive framework, organizations can not only forecast future trends but also prescribe optimal actions to mitigate risks and capitalize on market opportunities. This strategic application of data integration sets a new benchmark in supply chain efficiency, reducing operational friction and enhancing overall performance.
How Data Integration Transforms Supply Chain Operations
Data integration serves as a catalyst for elevating supply chain operations by unifying data from disparate sources into a single, cohesive ecosystem.
This transformation is about leveraging integrated data to drive operational excellence and strategic decision-making.
Companies that harness the power of data integration are poised to enhance efficiency, reduce costs, and create a more agile supply chain network.
Enhanced Demand Forecasting and Inventory Management
One of the most significant advantages of data integration is its impact on demand forecasting and inventory management.
By combining data from sales trends, market analytics, point-of-sale systems, and external market conditions, organizations can develop more accurate demand predictions. This integration minimizes the risks of overstocking or stockouts, ensuring that inventory levels are precisely aligned with market needs.
Retail giants like Walmart utilize integrated data analytics to anticipate spikes in demand during seasonal sales, allowing them to adjust inventory levels in real time and reduce the risk of stockouts or overstocking. This data-driven approach enhances supply chain responsiveness and improves customer satisfaction.
Optimized Logistics and Transportation
Data integration enables a real-time view of logistics operations, empowering companies to streamline their transportation and distribution processes. By integrating GPS data, traffic patterns, weather updates, and warehouse status, businesses can dynamically optimize delivery routes and schedules, reducing transit times and fuel costs.
Companies in the e-commerce sector, such as Amazon, use integrated data to optimize their delivery logistics. By analyzing traffic data and using predictive models, they can adjust delivery routes to ensure faster shipping and reduced logistics costs.
Predictive Maintenance and Asset Utilization
Integrated data plays a pivotal role in predictive maintenance by analyzing real-time sensor data from manufacturing equipment and logistics assets. Machine learning algorithms can identify patterns indicating potential failures, allowing organizations to proactively address issues before they lead to costly downtimes.
General Motors (GM) has been at the forefront of leveraging IoT and AI technologies for predictive maintenance across its global manufacturing plants. By integrating real-time sensor data with AI algorithms, GM is able to monitor the health of its machinery and identify potential failures before they occur, significantly reducing unplanned downtime and operational costs.
Supplier and Procurement Optimization
Data integration transforms supplier and procurement processes by providing a comprehensive view of supplier performance, market rates, and production schedules.
Organizations can make more informed sourcing decisions and foster stronger supplier relationships by aligning procurement strategies with real-time data insights.
Samsung Electronics, a global leader in the electronics industry, uses an advanced data integration platform to track supplier performance, production schedules, and market prices. The platform provides real-time insights, enabling Samsung to adjust sourcing decisions dynamically.
This agility in procurement has allowed Samsung to navigate supply chain disruptions effectively, ensuring that production remains on track and inventory levels are optimized, even during global component shortages.
Data integration is not merely a technical upgrade, it is a strategic enabler that transforms the way supply chains operate. By creating a unified data ecosystem, organizations can turn insights into action, driving a more responsive and resilient supply chain.
Increased Customer Satisfaction and Responsiveness
Seamless data integration enhances customer experience by enabling faster response times, more precise order fulfillment, and greater transparency. When supply chain data is integrated, companies can quickly adapt to changing customer demands and deliver products more efficiently, leading to improved satisfaction and loyalty.
Leading e-commerce platforms use integrated supply chain data to track order status in real time, providing customers with accurate delivery updates and swift resolutions to issues. This level of responsiveness not only meets customer expectations but also builds trust and fosters long-term relationships.
Data integration transforms supply chains from reactive systems into agile, proactive networks capable of delivering superior performance.
Best Practices for Successful Data Integration in Supply Chain
As global supply chains become more complex and data-intensive, leading companies are no longer relying on outdated methods to stay competitive. They’ve evolved, incorporating AI-driven data integration strategies to gain real-time insights and predictive power over their operations.
Companies that have embraced these technologies are slashing costs, optimizing workflows, and staying agile in an unpredictable market. Those still clinging to manual processes or basic analytics risk being outpaced by competitors who are using AI to make smarter, faster, and more accurate decisions.
In the world of modern supply chains, failing to integrate AI means falling behind. For supply chains to truly benefit from data integration, implementing AI-driven strategies is essential.
Here are three best practices that emphasize the integration of AI into supply chain data systems:
Prioritize AI-Enhanced Data Integration Platforms
Choosing the right data integration platform is fundamental to scaling supply chain capabilities. AI-enhanced platforms enable real-time data analysis and transform raw information into actionable insights.
These platforms should be designed to handle complex data structures, ensuring that they can adapt to the growing demands of supply chain networks.
Tools like Microsoft Azure Synapse Analytics, IBM Watson Supply Chain, and Tableau are commonly used in supply chain operations for their advanced AI and machine learning capabilities.
These platforms facilitate seamless data integration and provide predictive analytics that help optimize demand forecasting, inventory management, and logistics planning.
Focus on Machine Learning for Predictive Analytics
Machine learning (ML) algorithms are at the heart of maximizing the value of integrated data. By embedding ML models into the data integration framework, supply chains can predict disruptions, optimize procurement, and streamline logistics operations.
This predictive approach allows organizations to act swiftly in response to market changes, enhancing agility and reducing risks.
Foster Cross-Functional Collaboration
Successful data integration isn’t just about technology, it also requires fostering collaboration across different departments. Bringing together supply chain managers, data scientists, IT experts, and business leaders ensures that AI-driven insights are utilized across the organization.
This collective approach aligns data integration strategies with broader business goals, enhancing overall performance.
Cross-functional collaboration helps in creating a unified data culture, where all stakeholders have access to real-time insights, driving faster and more informed decision-making throughout the supply chain.
By prioritizing these best practices, organizations can build a resilient data integration strategy that not only drives efficiency but also empowers innovation and sustained competitive advantage.
Case Study:
TADA Cognitive Solutions

The Solution
TADA’s Supply Chain Control Tower was deployed to address these challenges by integrating data across the supply chain in real time. The technology provided comprehensive end-to-end visibility, allowing the manufacturer to track and optimize the flow of goods from suppliers to manufacturing plants and distribution centers.
Key features of TADA’s data integration approach included:
-
AI-Enabled Digital Twin Technology:
Used to simulate the supply chain operations in real-time, providing a predictive view of supply chain dynamics and enabling proactive decision-making. -
Enhanced Supply Chain Visibility:
Improved transparency at every stage, from inventory management to logistics, allowing for more accurate demand forecasting and supply planning. -
Data-Driven Insights:
Provided actionable analytics that helped the company reduce inventory by 20% and cut expedited costs by $10 million.
This case study exemplifies how advanced data integration and AI-driven insights can dramatically enhance supply chain performance, leading to substantial cost savings and improved operational efficiency.
Key Takeaways to Build a Data Strategy That Works
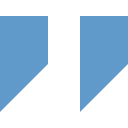
The integration of data in supply chain operations is no longer just a competitive advantage, it is a fundamental requirement for companies striving to lead in a rapidly evolving market landscape.
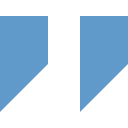
As demonstrated by the case studies and best practices outlined in this article, AI-powered data integration not only drives cost efficiencies and operational agility but also enhances real-time decision-making and customer satisfaction.
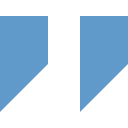
For companies aiming to stay ahead, the journey towards data integration involves more than just adopting new technology. It requires a strategic alignment of people, processes, and AI-powered tools to extract the full value of their data assets.
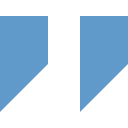
As businesses continue to grow in complexity and market demands increase, embracing data integration and AI-driven solutions will be critical to building resilient, future-proof supply chains that can adapt to any disruption.
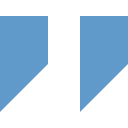
The future of supply chain management lies in the ability to anticipate, optimize, and innovate. All of this is made possible by the intelligent use of integrated data. As the industry continues to evolve, those who harness the power of data will set the standard for efficiency, agility, and customer satisfaction.
Frequently Asked Questions
What is data integration in supply chain management?
Data integration in supply chain management involves consolidating data from multiple sources, such as ERP systems, IoT sensors, CRM platforms, and logistics databases, into a unified framework. This process enables companies to have a holistic view of their supply chain operations, leading to better decision-making, improved efficiency, and reduced costs.
How does data integration improve supply chain performance?
Data integration enhances supply chain performance by providing real-time visibility into operations, enabling predictive analytics, and streamlining logistics and inventory management. By integrating data across all touchpoints, organizations can reduce lead times, optimize routes, manage inventory more effectively, and respond proactively to disruptions.
What are the benefits of AI-driven data integration in supply chains?
AI-driven data integration provides advanced capabilities like predictive analytics, adaptive decision-making, and automated processes. These tools help businesses forecast demand, optimize logistics routes, reduce operational costs, and make data-driven decisions faster. AI platforms like Kinaxis RapidResponse and TADA Cognitive Solutions have shown significant improvements in supply chain efficiency through real-time data integration.
What are the common challenges in implementing data integration in supply chains?
Implementing data integration in supply chains can be challenging due to issues like data silos, inconsistent data quality, integration with legacy systems, and resistance to change from stakeholders. Addressing these challenges requires robust data governance protocols, scalable AI platforms, and a clear strategy for aligning technology with business objectives.
How does predictive analytics play a role in modern supply chains?
Predictive analytics leverages historical data, machine learning algorithms, and AI to forecast future trends in supply chains, such as demand fluctuations, potential disruptions, and market changes. This capability allows organizations to make proactive decisions, optimize inventory levels, reduce lead times, and improve customer satisfaction by anticipating issues before they arise.